Synaptic electronics: From materials properties to next-generation memory devices
Phase-change memories (PCMs) are envisaged as one of the most promising solutions for neuromorphic computing in the semiconductor industry. These non-volatile memory devices rely on reversible switching of the electrical conductivity upon crystalline-to-amorphous phase change in chalcogenide compounds, typically Ge-Te based systems. This technology is criticized by the large power consumption associated with the phase transition. The success story builds on this limitation. It aims an interoperable multi-physics, multi-model, multi-equation, hierarchical and scale-reversible workflow solution for the investigation of material at device level, in which the materials characteristics are inherently connected to the device performance.
The project partners, CNR and Applied Materials®, are developing a predictive tool for industrial stakeholders that aims to optimize the device efficiency. To achieve this, they identify a direct relationship between the properties of materials (e.g. composition, stoichiometry, local order, defect) and the electrical performance of PCMs. They focus on the alloying effect due to the inclusion of elements such as As, Se, and Si on the switching and electrical response of GeTe compounds in the specific PCM configuration.
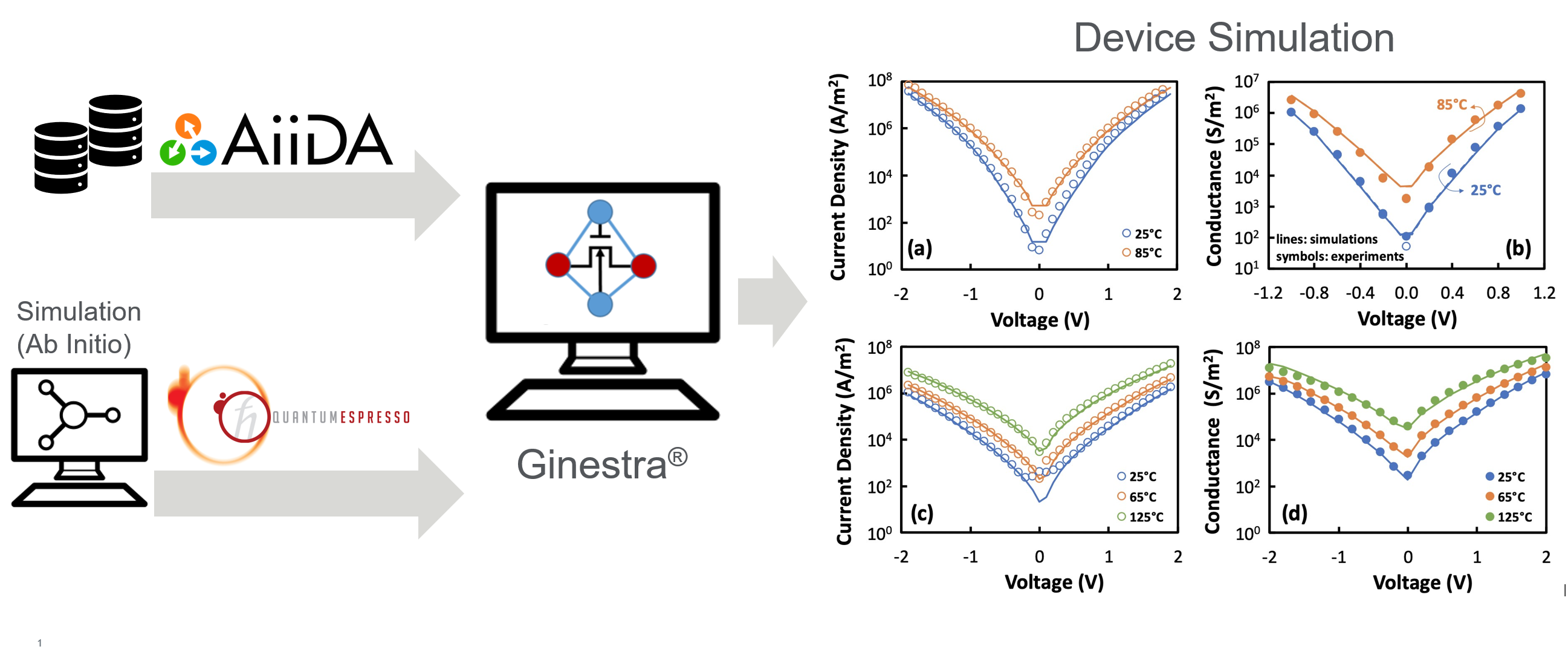
Synaptic electronics workflow. Data source Deliverable 3.2 INTERSECT project.
The interoperable interconnections of original third-party models (DFT, device) and tools (engine codes: Quantum ESPRESSO (QE), and Ginestra®) through the Open Model OIP serve the core technology of this use case. Shortly explained, QE is a software tool for electronics and atomistic models, based on DFT, for quantum mechanical materials modelling and Ginestra is an Applied Materials proprietary commercial software for atomistic and continuum models, specifically oriented to advanced device simulation. Most relevant workflows are controlled by AiiDA and associated to automatic pipes for the evaluation of the material quantities needed for the device.
KPIs
- Cost reduction
- Time to market acceleration
- Reduction of experimentation activity (number of samples)